RESEARCH PRESENTATION - aMIR SHIRDEL
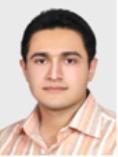
Robust System Identification
My research focuses on using support vector machines for identification of a dynamical system from experimental data, which forms an important problem in various control and signal processing tasks. Support vector Regression is a promising linear and nonlinear modeling method that has been found to perform very well in many fields, and has a powerful potential to be applied in system identification.
An important part of my research consists of developing identification methods for hybrid and switching systems, for which efficient general identification methods are largely lacking, especially for nonlinear systems.
The work is planned to include the following topics:
- Robust system identification using Laguerre and/or Kautz filter expansions and support vector regression
- Support vector regression for identification of Hammerstein models
- Identification of switching systems using support vector regression
More Information
Identification of a model of a dynamical system from experimental data forms an important problem in various control and signal processing tasks. As identified models are always uncertain, a particular problem arising in system identification is to ensure that the model does not only describe the specific data used in the identification, but that the model error is bounded as well.
Support vector machines (SVMs) [1] have been shown to provide a powerful method for robust classification and regression. This research will focus on a number of open problems in robust identification of dynamical systems using support vector regression. The work is planned to include the following topics.
- Robust system identification using Laguerre and/or Kautz filter expansions and support vector regression (SVR). Although robust system identification is not a new problem and has been studied before, SVR provides a natural way to incorporate bounds on the norm of the model uncertainty, which is not invalidated by the data, and to minimize this bound for various types of disturbances influencing the system. The computations can be performed efficiently, as special purpose methods exist for solving SVR problems. This work will in part be a continuation of [2].
- Support vector regression for identification of Hammerstein models. SVR is particularly well suited for black-box identification of nonlinear systems, as it leads to a convex problem for which convergence to the optimal solution can be guaranteed. A particular feature of nonlinear dynamical systems is that they can be represented using structures in which the nonlinearities and dynamical components are described by separate blocks. The most common system structures are the Wiener [3] and Hammerstein models. The purpose of the study is to develop SVR methods to identify Laguerre/Kautz Hammerstein models.
- Identification of switching systems using support vector regression. Switching systems have the property that their dynamical behavior may switch between a number of different modes. Identification of switching systems consists of identifying both the individual models, which describe the systems in the various modes, as well as the time instants when the mode switches have occurred. This problem is considerably harder than standard identification of a single model, and no efficient method for identification of switching systems exists today, especially for nonlinear systems. However, support vector regression has features which make it very promising for this problem, including identification of nonlinear switching systems.
Some references
[1] Vapnik, V.: ‘The nature of statistical learning theory’ (Springer-Verlag, New York, 1995)
[2] Tötterman, S., and H. T. Toivonen: ‘Smoothness priors support vector method for robust systems identification’, IET Control Theory & Applications 3 (2009), 509-518.
[3] Tötterman, S., and H. T. Toivonen: ‘Support vector method for identification of Wiener models’, J. Proc. Control 19 (2009), 1174-1181.